Unlocking the Power of Synthetic Data for Deep Learning

In the rapidly evolving field of artificial intelligence (AI),deep learning has emerged as a powerful technique for solving a wide range of complex problems. However, the effectiveness of deep learning models heavily relies on the availability of high-quality data for training. In many real-world scenarios, acquiring sufficient real-world data can be challenging due to factors such as privacy concerns, data scarcity, or the cost and effort of data collection.
5 out of 5
Language | : | English |
File size | : | 67764 KB |
Text-to-Speech | : | Enabled |
Screen Reader | : | Supported |
Enhanced typesetting | : | Enabled |
Print length | : | 689 pages |
Synthetic data offers a compelling solution to this challenge. It involves generating artificial data that mimics the characteristics and distribution of real-world data. By leveraging synthetic data, researchers and practitioners can significantly expand their training datasets, improve model performance, and overcome data limitations.
In this comprehensive guide, we will delve into the transformative potential of synthetic data for deep learning. We will explore the latest advancements, applications, and best practices to empower your AI projects. By the end of this guide, you will gain a deep understanding of the following:
- The fundamentals of synthetic data generation and its benefits for deep learning
- Different techniques for generating synthetic data, including generative adversarial networks (GANs) and variational autoencoders (VAEs)
- Applications of synthetic data in various domains, such as self-driving cars, healthcare, and natural language processing
- Best practices for evaluating the quality of synthetic data and ensuring its effectiveness for deep learning
- Emerging trends and future directions in synthetic data research
The Rise of Synthetic Data in Deep Learning
The growing popularity of synthetic data in deep learning can be attributed to several key advantages it offers:
- Data Augmentation: Synthetic data can be used to augment existing real-world data, increasing the size and diversity of the training dataset.
- Data Privacy: Synthetic data can be generated while preserving the privacy of individuals, addressing concerns related to data sharing.
- Cost and Time Savings: Generating synthetic data is often more cost-effective and time-efficient compared to collecting real-world data.
- Control over Data Distribution: Synthetic data allows you to control the distribution and characteristics of the data, ensuring it aligns with the specific requirements of your deep learning model.
Techniques for Synthetic Data Generation
There are various techniques available for generating synthetic data. Some of the most commonly used methods include:
- Generative Adversarial Networks (GANs): GANs are a type of generative model that can learn the distribution of real-world data and generate new samples that resemble the original data.
- Variational Autoencoders (VAEs): VAEs are another type of generative model that can be used to generate synthetic data by learning a latent representation of the original data.
- Sampling from Existing Distributions: In some cases, synthetic data can be generated by sampling from known distributions that closely resemble the real-world data.
Applications of Synthetic Data in Deep Learning
Synthetic data has found wide-ranging applications in various domains of deep learning:
- Self-Driving Cars: Synthetic data can be used to train self-driving cars on a vast range of scenarios, enhancing their safety and performance.
- Healthcare: Synthetic data can be used to train deep learning models for medical diagnosis, drug discovery, and patient monitoring, improving healthcare outcomes.
- Natural Language Processing (NLP): Synthetic data can be used to train NLP models on large datasets of text and speech, enhancing their understanding and generation capabilities.
- Computer Vision: Synthetic data can be used to train computer vision models on a wide variety of images and videos, improving their object detection, classification, and segmentation capabilities.
Best Practices for Evaluating Synthetic Data
To ensure the effectiveness of synthetic data for deep learning, it is important to evaluate its quality carefully. Some key best practices for evaluating synthetic data include:
- Quantitative Metrics: Using metrics such as accuracy, precision, recall, and F1 score to assess the performance of deep learning models trained on synthetic data.
- Qualitative Analysis: Manually inspecting synthetic data to identify any noticeable differences from real-world data and ensure it captures the relevant characteristics.
- Data Distribution Analysis: Comparing the distribution of synthetic data to the distribution of real-world data to ensure they align closely.
Emerging Trends and Future Directions
The field of synthetic data for deep learning is continuously evolving, with new research directions emerging:
- Semi-Synthetic Data: Combining synthetic data with real-world data to enhance the realism and effectiveness of deep learning models.
- Transfer Learning: Transferring knowledge learned from synthetic data to real-world data to improve model performance.
- Meta-Learning: Using synthetic data to train deep learning models that can adapt to new tasks quickly.
Synthetic data has emerged as a transformative tool in the field of deep learning. By enabling the generation of high-quality artificial data, synthetic data empowers researchers and practitioners to overcome data limitations, enhance model performance, and tackle a wider range of complex problems. As synthetic data research continues to advance, we can expect even more groundbreaking applications and applications in various industries.
To further explore the potential of synthetic data for deep learning, we highly recommend the comprehensive book "Synthetic Data For Deep Learning: Springer Optimization And Its Applications 174."
This book provides an in-depth analysis of synthetic data generation techniques, applications, and best practices. It is an invaluable resource for researchers, practitioners, and anyone interested in leveraging the power of synthetic data to drive innovation in deep learning.
5 out of 5
Language | : | English |
File size | : | 67764 KB |
Text-to-Speech | : | Enabled |
Screen Reader | : | Supported |
Enhanced typesetting | : | Enabled |
Print length | : | 689 pages |
Do you want to contribute by writing guest posts on this blog?
Please contact us and send us a resume of previous articles that you have written.
Book
Novel
Page
Chapter
Text
Story
Genre
Reader
Library
Paperback
E-book
Magazine
Newspaper
Paragraph
Sentence
Bookmark
Shelf
Glossary
Bibliography
Foreword
Preface
Synopsis
Annotation
Footnote
Manuscript
Scroll
Codex
Tome
Bestseller
Classics
Library card
Narrative
Biography
Autobiography
Memoir
Reference
Encyclopedia
David S Reynolds
Darren Kirby
David Olsen
David T Courtwright
David Batchelor
Deb Aronson
Danny Fingeroth
Debi Gliori
Deb Vanasse
Darren West
Daniel R Montello
David Domeniconi
David Canford
Danielle Krysa
David Ramsay
David Sloan Wilson
Dave Clayton
Dawn M Mcbride
Danny Peary
David E Hoffman
Light bulbAdvertise smarter! Our strategic ad space ensures maximum exposure. Reserve your spot today!
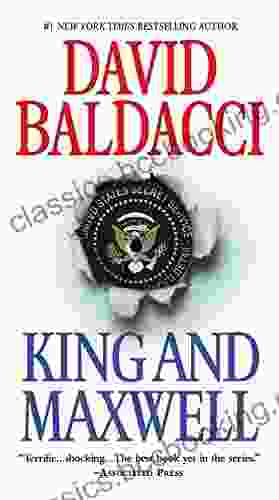

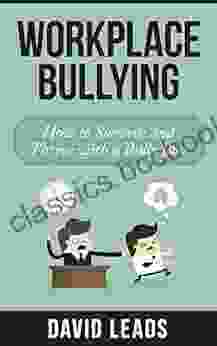

- Brayden ReedFollow ·14.8k
- Stuart BlairFollow ·5.5k
- Mike HayesFollow ·17.6k
- Jamie BlairFollow ·12.6k
- Aubrey BlairFollow ·17.2k
- Anthony BurgessFollow ·11.1k
- Davion PowellFollow ·4.2k
- Benjamin StoneFollow ·19.5k
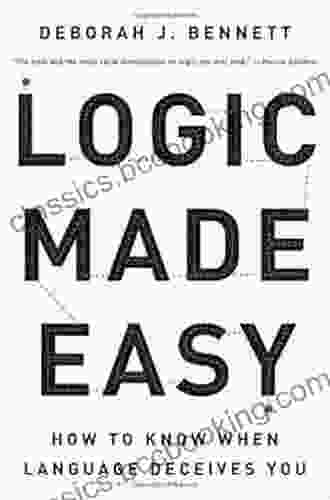

How to Know When Language Deceives You
Unmasking the Power of...
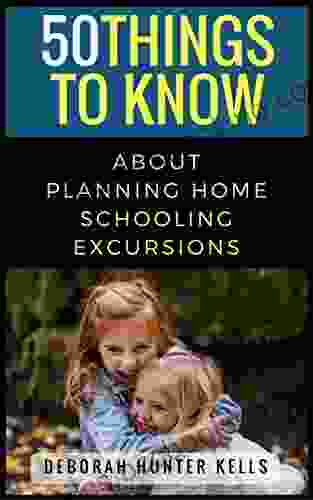

50 Things To Know About Planning Home Schooling...
: The Power of Hands-On Learning Embarking...
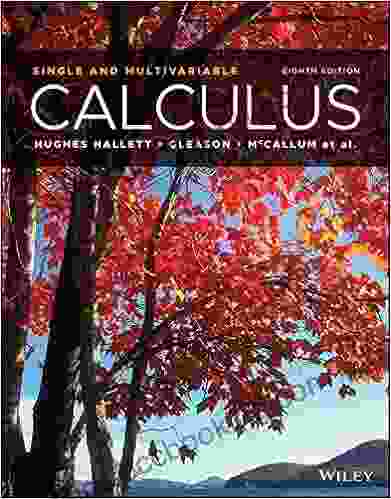

Calculus: Single and Multivariable, 8th Edition — The...
Calculus is the...
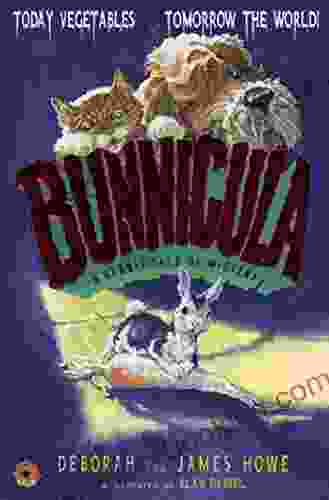

Bunnicula and Friends: A Spooktacular Tale of Mystery and...
In the quaint little town of Celeryville,...
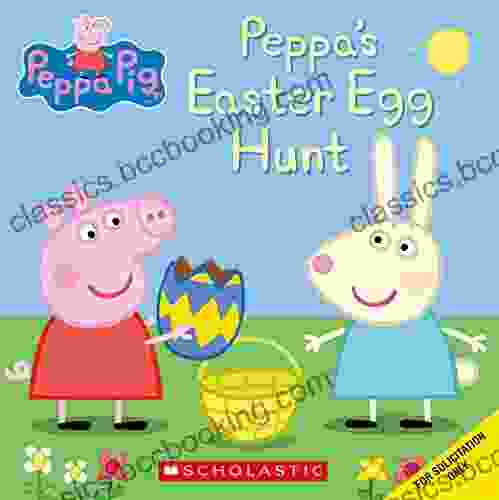

Peppa Easter Egg Hunt: Join Peppa Pig on an...
Get ready for...
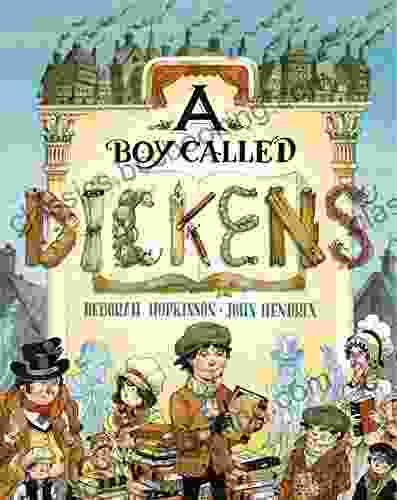

Boy Called Dickens: A Journey into the Childhood of a...
Delving into the...
5 out of 5
Language | : | English |
File size | : | 67764 KB |
Text-to-Speech | : | Enabled |
Screen Reader | : | Supported |
Enhanced typesetting | : | Enabled |
Print length | : | 689 pages |